Learn how test engineers at Kautex Textron use self-learning models to more accurately predict intractable fuel sloshing noise faster.
This webinar will showcase how the team at Kautex Textron was able to utilise Monolith’s machine learning capabilities to produce accurate AI models for predicting fuel sloshing behaviour. The methods developed helped engineers gain new and deeper insights into their complex products as well as create a toolchain that provides valuable predictions significantly faster than what state-of-the-art simulation tools such as Computational Fluid Dynamics (CFD) can provide.
In the session, we focus primarily on cost and time-critical test applications, and data-driven models are increasing ROI, without disrupting existing engineering workflows.
Who should watch?
What we will cover:
- How the engineers at Kautex Textron used the Monolith software to predict sloshing noise and design performance before actually performing physical testing, whilst dramatically accelerating product development.
- How Monolith’s self-learning models are instantly predicting the performance of highly nonlinear, intractable physical systems.
- How the Monolith platform leverages valuable engineering data and machine learning to rapidly find critical new insights hidden in historic and current test data.
Monolith leverages the power of self-learning models embedded in a no-code cloud environment to...
- Reduce R&D iterations by empowering designers to assess the performance of designs
- Perform a full exploration of multiple virtual test scenarios in one place
- Reduce costs and time investment for testing phases
- Allow for faster test cycles and performance insights
- Solve intractable physical problems
- Accelerate engineering R&D
Meet our speakers
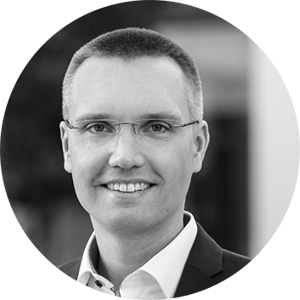
Dr. Bernhardt Lüddecke
Global Director of Validation, Kautex Textron
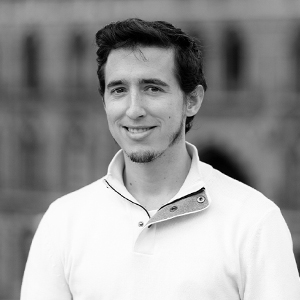
Dr. Joel Henry
Principal Engineer, Monolith
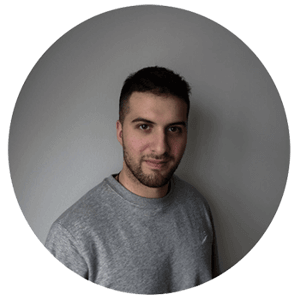
Jousef Murad
Product Marketing Engineer, Monolith