Industry
Empowering industrial engineers with AI
Monolith cloud-based AI software is built from the ground up for engineers with an intuitive, easy-to-use UI. It's designed for quick adoption and time to results for big data.
Get in touch with Monolith
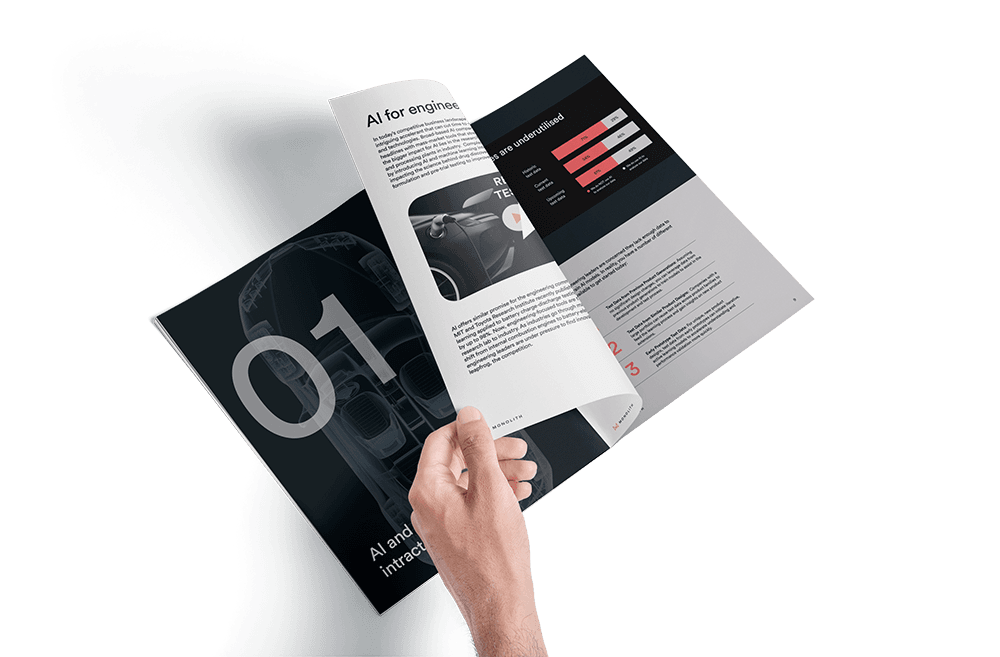
AI for simplifying validation testing
Four applications for AI in validation test
AI has a significant impact on validation testing in engineering product development. You can reduce testing by up to 73% based on battery test research from Stanford, MIT, and Toyota Research Institute. Learn more with Monolith.
“Monolith allowed us to understand and optimise the gas meter's behaviour for all operating conditions and optimise meter accuracy under extreme conditions, allowing us to build a superior, more accurate product in a much shorter amount of time.”
Dr. Bas Kastelein, Sr. Director of Product Innovation, Honeywell Process Solutions
Packaging optimisation using AI with AptarGroup
In this exclusive customer webinar, Fabio di Memmo from AptarGroup & Monolith CEO Richard Ahlfeld talk about the value of Monolith’s no-code AI platform for packaging, and how to accelerate decisions from months to minutes.
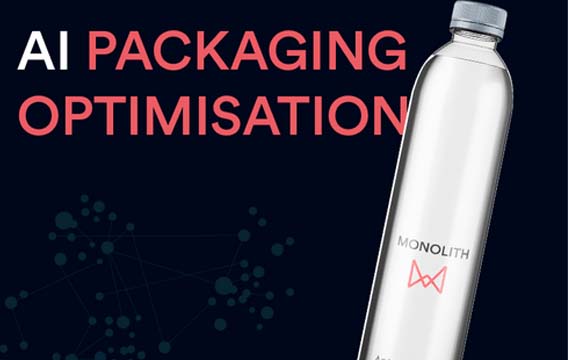
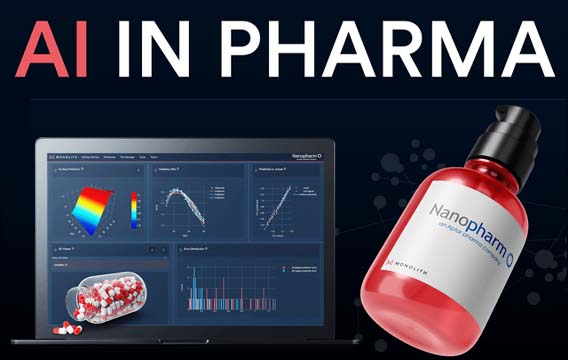
Optimising particle size and shape distribution from a target dissolution profile with Nanopharm
A random forest regression model was trained to predict the mass dissolved at different time points from the size and shape of the particles, to a good degree of accuracy. The resulting Monolith dashboards enabled users to upload a target dissolution profile and return an optimised particle size and shape distribution that would produce the target dissolution profile.
The next generation of smart meters using self-learning models
Using Monolith to investigate test data, users can combine, transform and build self-learning models inside our no-code AI platform that accurately predict flow rates for multiple material types. Devices such as valves with varying throughput capabilities including radius, length, and other relevant device measurements.
Common industrial engineering challenges
The time-to-market needs for industrial products and applications fail to meet customer expectations.
Exploring and understanding a design space for all potential use cases is time-consuming and an inefficient use of resources.
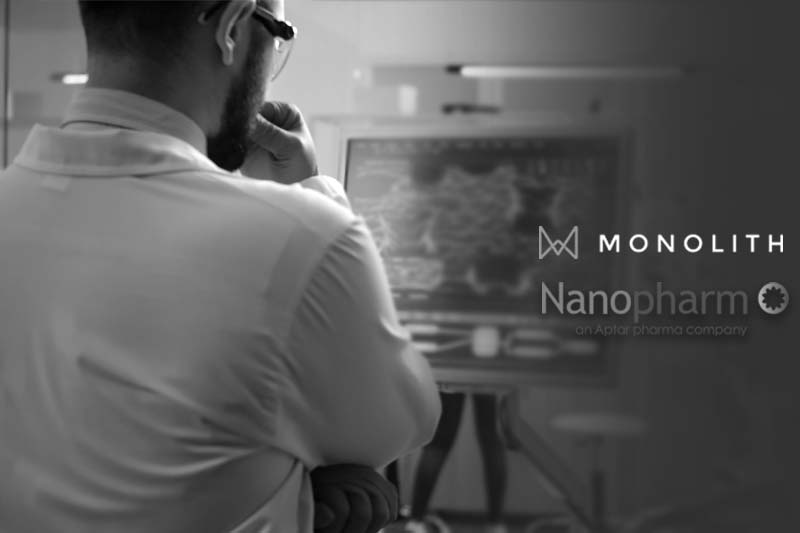
Case study
The next generation of pharmaceutical development using self-learning models
A random forest regression model was trained to predict the mass dissolved at different time points from the size and shape of the particles to a good degree of accuracy. The resulting Monolith dashboards enabled users to upload a target dissolution profile and return an optimised particle size and shape distribution that would produce the target dissolution profile.
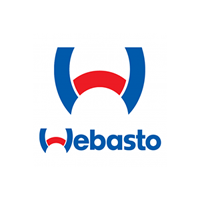
There are many potential use cases for AI to speed up the battery test and validation process. After an extensive evaluation, we found Monolith to be an excellent option for scaling AI across our R&D.
-Markus Meiler, VP Research & Development, Webasto
Resources