Case study
Packaging optimisation using AI | AptarGroup
The challenge:
While modelling and simulation are core tools in some industries, packaging manufacturers have failed to reap the benefits. Despite significant investment in model development and simulation, businesses such as AptarGroup, Inc found that the returns simply weren’t there. However, such organisations are data-rich, allowing them to consider a different approach using self-learning models.
The solution:
By leveraging the wealth of data generated during the testing of existing packaging designs, Monolith built a self-learning model to predict the performance of new designs. Because Monolith is AI software built by engineers for engineers, AptarGroup doesn’t need to involve data scientists and Python programmers. This leaves them able to improve the model themselves and even consider offering the power of AI to their customers.

The company:
AptarGroup, Inc serves markets as diverse as pharmaceutical, beauty, food and beverage, and personal care with differentiated packaging solutions. From pouches and pumps to sprays and dispensers, their market-driven innovations deliver breakthrough products that enhance brands and transform patients’ lives while spanning all market needs.
Modelling is common, but not democratised
In many industries, exploration of development approaches for a new product starts with creating a model. A mathematical model represents a physical solution that is imported into a simulation environment. From here, a multitude of test cases can be executed, allowing the design team to develop their understanding of the new product. Often, real-world data collected from measurements made on previous products can be used to improve the model. The obvious advantage is saving both time and money, as there is no need to build any physical prototypes until a handful of the most promising approaches have been determined.
However, access to simulation capability is not democratised. Aerospace is an example of a well-served industry. In fact, you can even fly an Airbus A320 from the comfort of your living room on your games console. But if you want to simulate a cardboard box, well, you’re out of luck. It turns out that such humble packaging is fiendishly difficult, if not impossible, to model.
Why is packaging so hard to develop?
Businesses such as AptarGroup, Inc are not ignoring the advances made using simulation tools in other industries. In fact, they’ve spent a significant amount of time and made considerable investments in modelling. Like everyone else, they are looking to optimise their design process and shorten their development cycles to remain innovative and nimble. However, the results have only led to disappointment. While significant progress has been made over the years, the resultant models haven’t delivered enough benefits to design teams.
Furthermore, like in every market, startups are chomping at the bit to get a piece of the around $900 billion worldwide packaging business pie. And, as we have seen, David sometimes wins against Goliath, despite the industry contacts, development team competency, and financial clout of big businesses.
Thankfully, this risk reduces as manufacturers discover the power of self-learning models using Monolith. Not only can businesses such as AptarGroup predict the improvements and failure modes of packaging designs, but they can also place that capability directly into the hands of their customers.
Today’s packaging development process
Packaging isn’t just a solution for storing a product. It often is the product, required to exude a brand’s values, underscore a lifestyle, and stand out again the competition. At the same time, it has to be functional. If, while unscrewing a bottle of water, the container collapses under your grip, spilling water all over your clothes, you’re unlikely to purchase it again. Or, if you knock it gently while reaching across a table, you’ll be unimpressed if the falling bottle gives your smartphone an unintended bath.
Design teams often require around two years to bring a new package to market, starting with assessing market demand, working through design concepts, and then testing the most promising designs. In fact, it turns out that the testing process is the key to developing self-learning models.
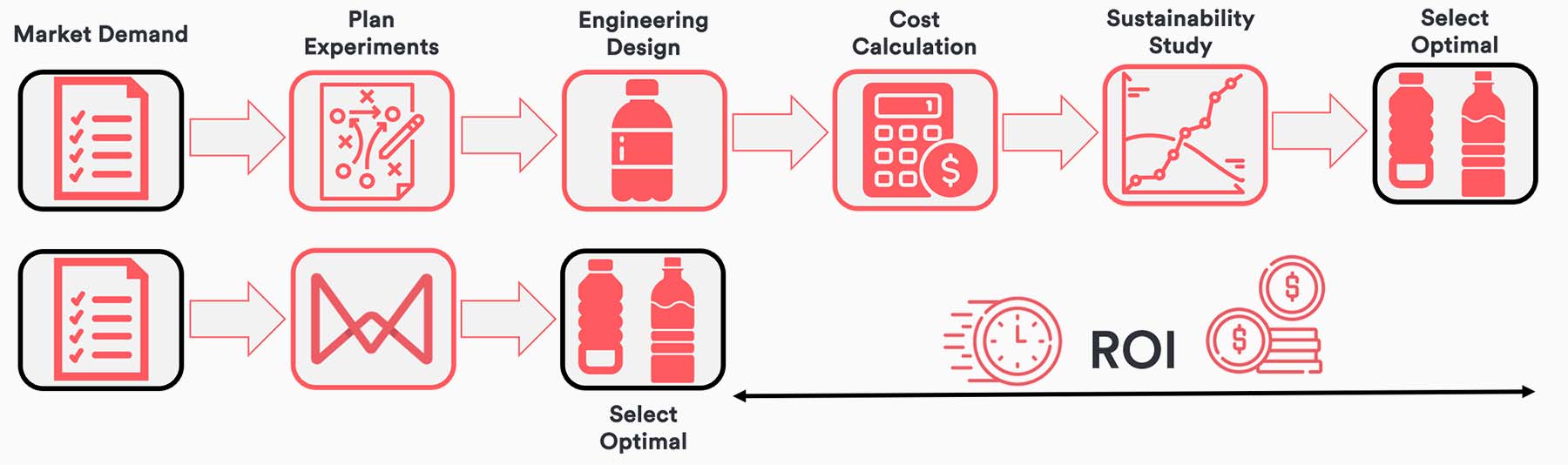
Self-learning models for improved bottle design
Bottles, filled to varying levels with liquids of different viscosities, are given nudges of varying forces to determine the conditions under which they fall over. This provides a significant number of data points for a wide range of input variables, something that is essential for analysis using AI.
This is a classic big-data problem and the point where the team at Monolith was able to demonstrate the power of self-learning models. By working alongside the AptarGroup team, Monolith engineers explained the optimal manner for applying AI to achieve a tangible return on investment (ROI). This started with examining past test results, including the bottle size, shape, material thickness, and liquid viscosity, and feeding it into a model. There was no need to work with data scientists or Python developers, something that typically requires a careful explanation of the data and an intimate understanding of the packaging business
Predicting performance without the ping-pong
From here, Monolith predicts how new bottle designs will respond to the nudge test. By setting up a dashboard, design engineers can adjust all the relevant design factors as well as the liquid properties and fill level. With a round of predictions completed, the team can review the stability of their new design ideas along with an assessment of the uncertainty in their results. AptarGroup’s designers remain in control of their test data and prediction tool, while also avoiding the delays commonly associated with working with data scientists as they wait for AI model improvements to be made. In addition, Monolith enables the team to test less while also learning more about the products they are developing.
The Future of Packaging Design
The beauty of Monolith’s self-learning models are their simplicity. Many businesses already have a wealth of data available that describes the current state of their products. Monolith leverages this data to determine how existing objects, such as bottles, work under various test conditions. This makes it a breeze to predict the test results for potential design changes, such as when the bottle wall is thicker, or the liquid is more viscous.
Such has been the success of Monolith in the packaging design process, AptarGroup is examining how direct access to the tool can be opened up to their customers. Today, those interested in a new package perform the same lengthy manual tests on existing packages as undertaken by suppliers. From here, they work with the supplier through the design process to optimise it to their needs. In the future, customers could use a Monolith-based portal to simulate their design changes in minutes, submitting their preferred design directly to the AptarGroup team.