Case Study
Predicting Sloshing Noise
& Reducing Testing With
Self-Learning Models
The team at Kautex-Textron was able to utilise Monolith’s self-learning model capabilities to produce
sufficiently accurate models for predicting fuel sloshing behaviour, and solved a previously intractable
physical problem combining existing acoustic test data & 3D CAD fuel tank shapes.
The Challenge:
Predicting fuel sloshing sound during vehicle deceleration and further is one of the most complicated, multi-physics challenges for engineering teams to model and understand.
The Solution:
The team at Kautex-Textron was able to utilise Monolith’s self-learning model capabilities to produce sufficiently accurate models for predicting fuel sloshing behaviour, and solved a previously intractable physical problem combining existing acoustic test data & 3D CAD fuel tank shapes.
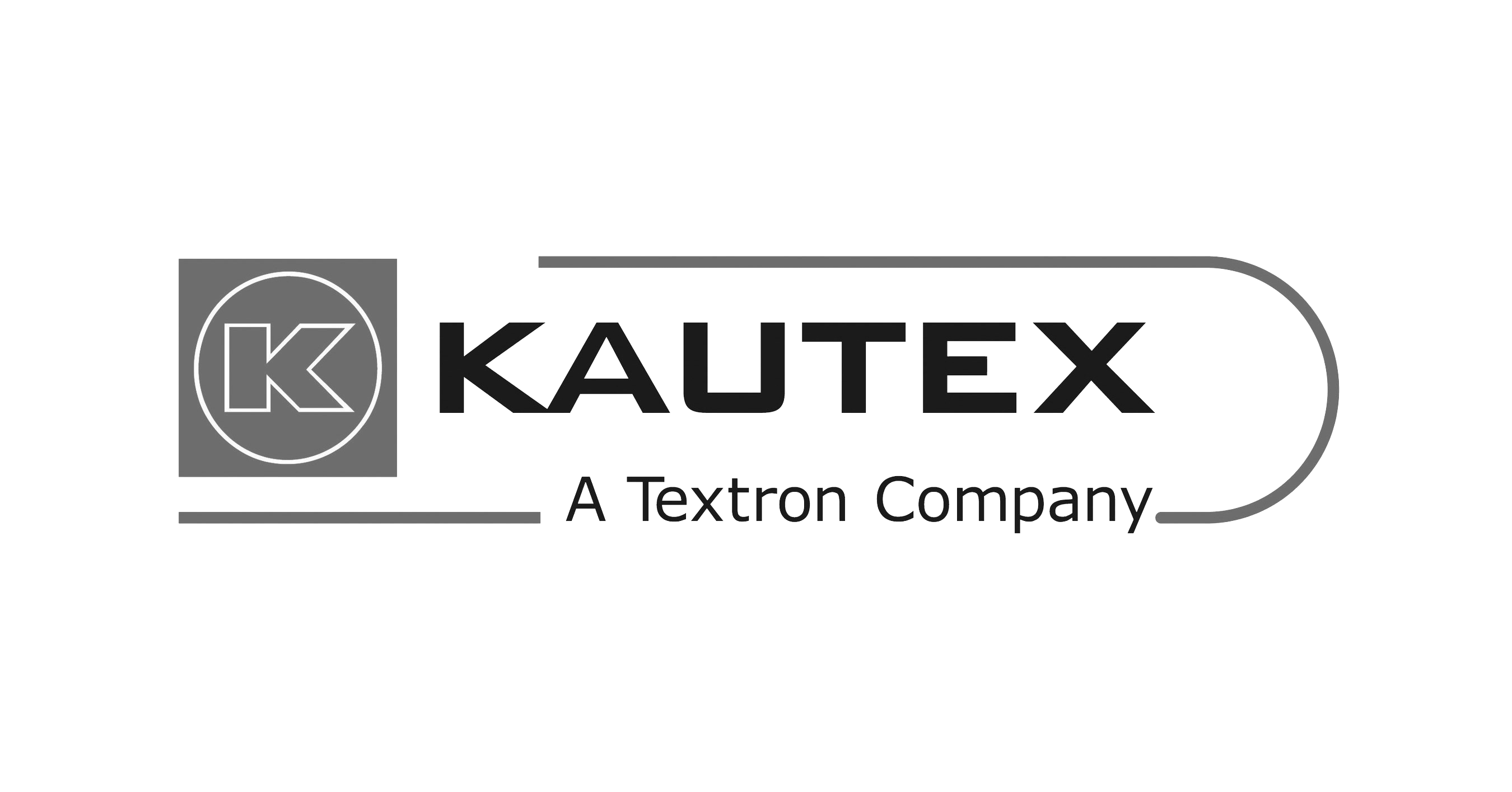
The Company:
At Kautex, we are driving the future. As a Tier One automotive supplier with more than 30 plants in 14 countries, Kautex designs, develops and manufactures traditional and hybrid fuel systems, advanced cleaning solutions for assisted and autonomous driving, engine camshafts and plastic industrial packaging solutions.
Background
Test campaigns are notoriously expensive for engineering companies owing largely to a heavy reliance on physical prototypes. One of the most pertinent uses of AI in engineering is to conduct “virtual testing”. One of the challenges with virtual testing using classical simulation methods is that they often rely on data that is very expensive to create in the first place.
Before Monolith, the engineers at Kautex heavily relied on those physics-based simulations as well as physical tests. The CFD approach was limited as the results were unable to fully match the fidelity of the experimental results.
Now, the Kautex engineers gain new and deeper insights into their complex products and created a toolchain using Monolith. That provides valuable predictions significantly faster than what state-of-the-art simulations can provide.
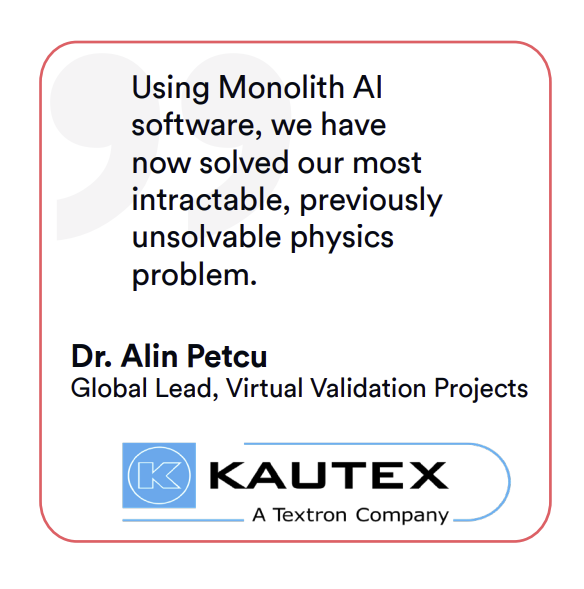
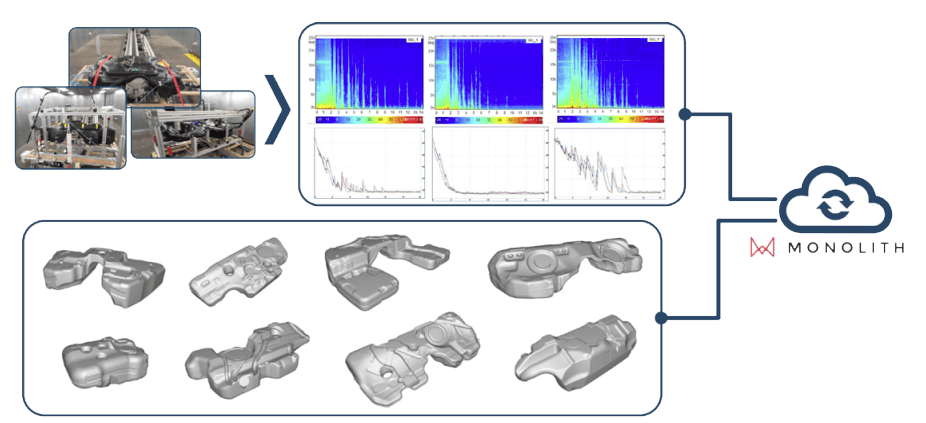
Physical test setup for the sloshing (top left), measured acoustic signals (top right) and 3D data (bottom) - all fed into the Monolith platform to solve an intractable physical problem.
Fulfilling Legal Requirements
Predicting fuel sloshing noise during vehicle deceleration and further is one of the most complicated, multi-physics challenges for engineering teams to model and understand. Using a data-driven approach, a previously unsolvable physics problem, can now be solved using the power and capabilities of self-learning models by combining the engineer’s expertise, acoustic data and Monolith’s platform. Self-learning models help to calibrate complex systems based on test data and 3D shape information to ultimately use less test data to more accurately calibrate a product across a range of operating conditions and to fulfill legal or customer requirements.
From CFD to Self-Learning Models
In the traditional simulation approach, engineers will always need to rely on noise indicator studies and physics-based simulations, whereas training AI models with full fletched measurement/test data enable a resolution of the full frequency to learn from using the data-driven approach.
Deployment of AI Solutions
Kautex’ Leadership team is working hard to help AI becoming a more integrated part of engineers’ day-to-day work. The Monolith platform offers multiple features that enable the sharing of results across teams and the entire organisation. Once the engineers complete a notebook where they build the pipeline to explore and manipulate the data, run AI models and investigate the results, they can easily create shareable dashboards in an elegant, simple and intuitive manner.
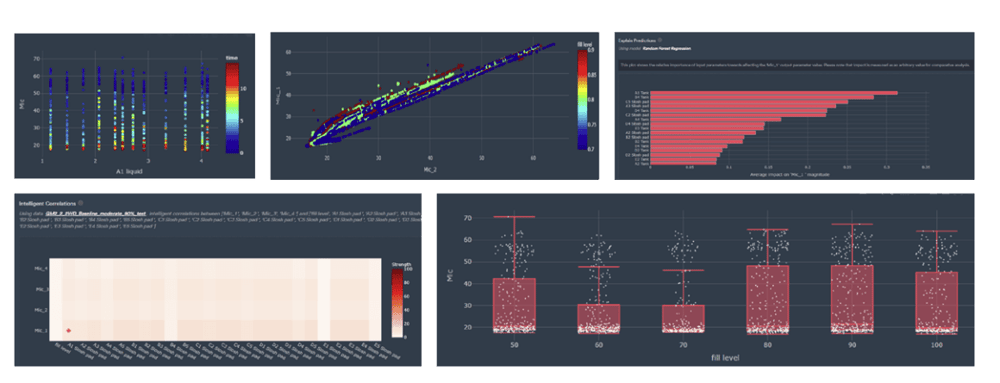
Data exploration features inside of Monolith allowed the Kautex engineers to investigate their large engineering datasets before manipulating them for
further processing.