Why ask this question? Simple. Vehicle track testing is expensive, and demand is growing. If an automotive OEM could reduce the need for a vehicle to circulate a test track by 20%, that would get management excited.
The addiction to track testing comes from a thirst for the “real-life” test data it provides. It supports everything from the development and prototyping of new vehicles, to endurance testing, to performing a comparison with competitor vehicles.
Demand for expanding track testing
The demand for track testing is growing. It’s being fuelled by the increasing complexity of vehicle testing and a surge in the launch of new models.
The trend towards autonomous driving and advanced driver assistance systems (ADAS) means there is lots more to test. In 2014, the Euro NCAP assessment included 6 ADAS tests, and by 2020 there were 39 ADAS tests -an increase of 650%!
The switch to EV’s and consumer’s desire for niche vehicles means there are more new models under development than ever. The Renault Group unveiled in January of this year a major new strategic plan, the “Renaulution”, which includes the introduction of 24 new vehicles by 2025.
When you bring together an increasing complexity of vehicle testing and more new vehicles to test -no surprise that demand for track testing goes up. GM’s new facility in Ontario, Canada, opened earlier this year specifically for Self-Driving and EVs. BMW has similarly just built a facility in the Czech Republic for Self-Driving and EV’s that cost €100m. As I said -this is an expensive business.
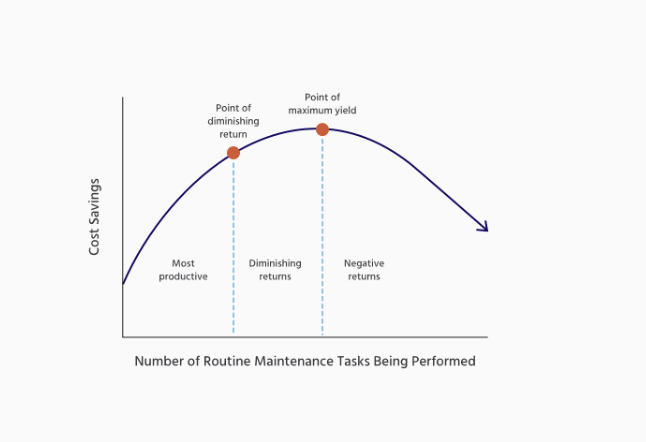
Track testing itself is very structured and highly sophisticated. Vehicles are covered in hundreds of sensors, and the test driver performs an array of predetermined tests. Everything from driving over different road surfaces to performing specific driving manoeuvres in a “mock” urban setting. But you can’t test every combination of variables -it’s too expensive and time-consuming. It’s a game of diminishing returns.
And there is one significant variable that can’t be controlled -air temperature. Changing the temperature from 29.5C to 0C can increase the density of the air by 11%. So theoretically, the engine would create 11% less power. That will have a significant impact on the vehicle's performance such as how the car feels whilst accelerating, cornering forces, etc. It’s why OEM’s use warm weather tracks and cold weather tracks. But with the growing impact of climate change, you can never guarantee the weather you’ll encounter in Southern Spain in June or Northern Sweden in January.
This leaves us with OEMs spending huge amounts of time and money on track testing yet they don’t walk away with a complete understanding of vehicle performance. Those gaps in knowledge can hurt. Everything from poor reviews to a delay in the launch date.
Can AI help measure your tests?
Interestingly, in 2019 the Capgemini’s Research Institute identified a number of “high-benefit” AI use cases for the automotive industry. Predicting the outcome of track testing was one of them.
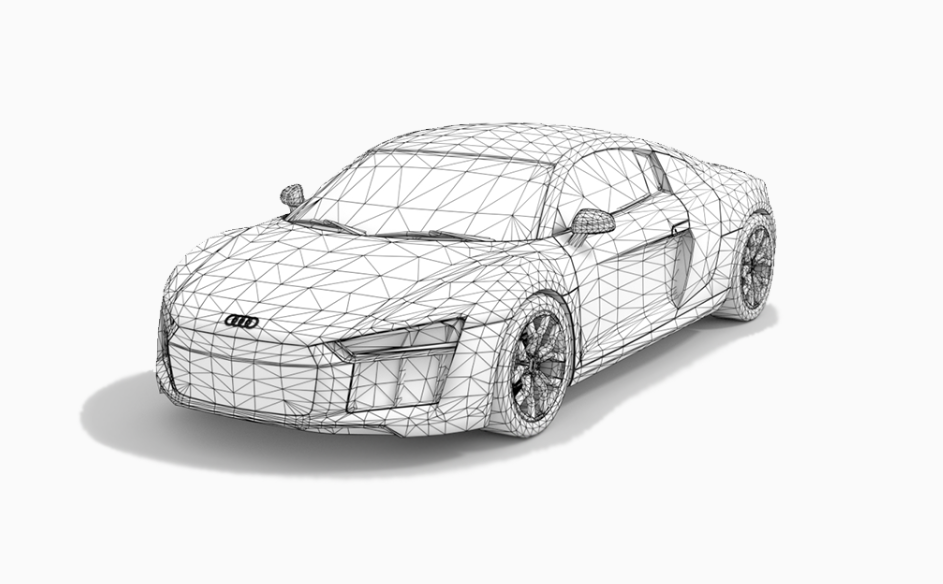
Before introducing MonolithAI and sharing our experiences with track testing; it’s worth answering the question, “what is AI?”. It means different things to different people.
According to McKinsey, AI is a collective term for the capabilities shown by learning systems that are perceived by humans as representing intelligence. AI gives machines the ability to “think” and act, in a way that previously only humans could. Today, typical AI capabilities include fraud detection, image and video recognition, bots/conversational agents, and the recommendation engines of the likes of Amazon.
Critical to the accuracy of the prediction the AI model makes is the volume of good-quality test data that is available to train it. And the data needs to include a complete range of input parameters and output parameters. So, to predict the dynamic behaviour of a vehicle, input variables are the steering angle and the pedal actuations, whilst output variables are the forces exercised on the front-right wheel, for example. With hundreds of sensors attached to the vehicle and data being transmitted every tenth of a second potentially -track testing creates lots of good quality data.
This type of data helps OEMs paint of a picture of how a complex suspension system performs across a range of driving scenarios. And with AI, they would require far less testing to establish this understanding for a new car -that is the theory!
What’s Monolith AI’s experience with vehicle tracking testing?
Monolith is an AI platform specially designed for testing simulations and testing workflows that an engineer might face. It’s a tool that happily consumes lots of different formats of engineering data from the testing track and beyond, and customers include the likes of Honda and BMW.
Just about the first question engineers ask us is, “how do I trust the results, the predictions?” which is natural; engineers always look to validate their results.
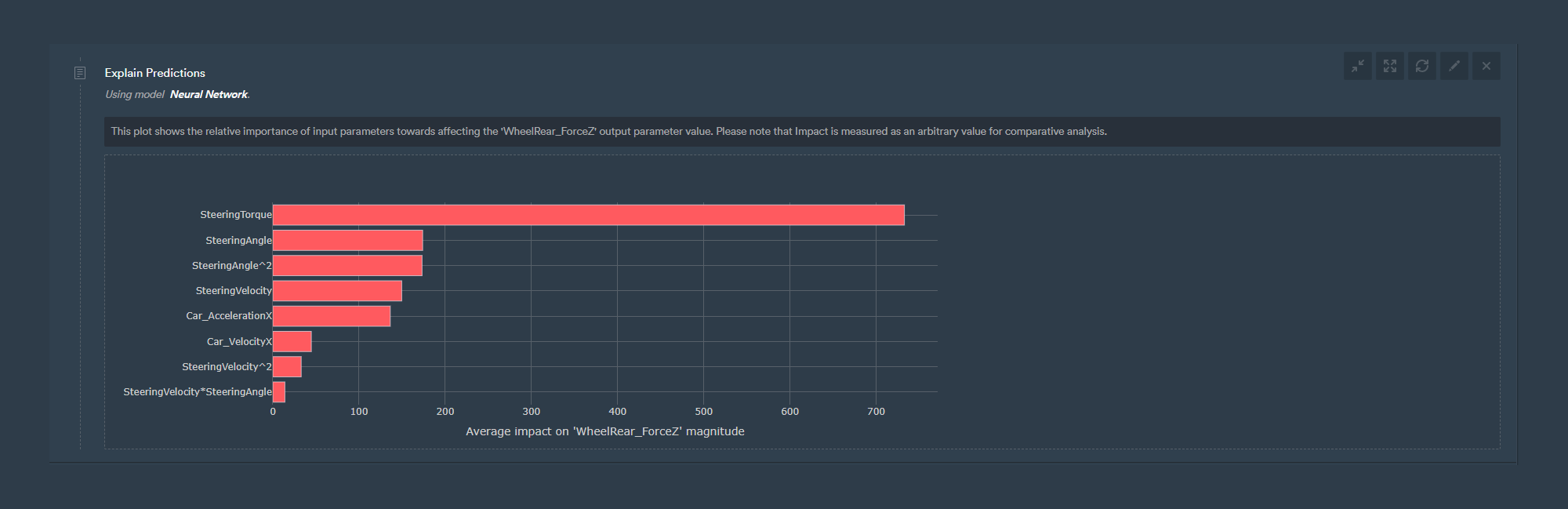
In the world of AI, Monolith has extensive visualisation capability designed to increase testing understanding, quantify test accuracy, and support team collaboration.
This “explain prediction” is a great example. It shows a ranking of the most important testing input parameters in affecting the output we are interested in. Below, you can see the vertical force exerted on the wheel during steering manoeuvres.
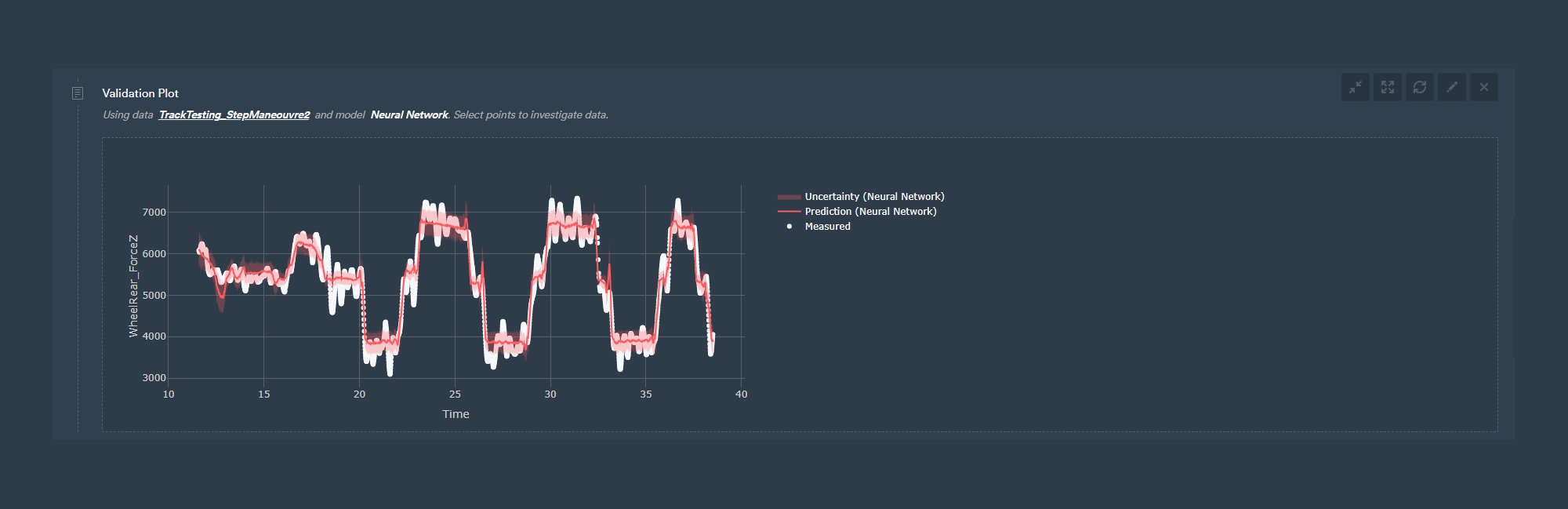
The Monolith AI platform allows a user to test the accuracy of their AI model by performing a “test, train split”. Typically, 80% of the original test data is used as input to train the AI model and 20% to test it -to evaluate the accuracy of the model.
When performing the test, you only share the input data with the model -not the output. Then, looking at the plot, you see the accuracy of the model over the entire design space. The red line is the predicted output parameter and white, actual. By hovering over any point -you see the accuracy level expressed as a percentage.
Witnessing how the engineers leveraged the Monolith AI software platform during track testing was very insightful. Rather than spending the day working through a series of predetermined test campaigns, the engineers used Monolith to perform and create real-time test campaign optimisation.
That means they would add the testing data collected in their first campaign to their mountain of historical data from different vehicles and ask Monolith to run a prediction. This would highlight areas of volatility in the results and where the results did not meet the required accuracy level. These areas would be the focus of the next test campaign.
The engineers were running test campaign iterations with AI in the loop to make more informed decisions. The impact was significant.
One Monolith user witnessed a 70% reduction in track testing time in one case and a corresponding 45% reduction in cost.
You should be aware that there are a number of factors that influence the size of the reduction in track testing time our customers have reported back to us.
These include the type of track test (endurance-focused versus a new car prototype, for instance), the level of accuracy the engineers required, the size of the envelope (number of variables and range of each variable) the track test was looking at, etc.
One extra point to highlight. I mentioned earlier the significant impact that air temperature has on the vehicle’s performance. It’s one of the very few things outside the control of the vehicle dynamics team. One engineer said:
“I was really impressed with how the Monolith AI platform harvested all our historic track test data to predict how car “X” would perform at 20C when we’d only tested it 25C. It seems obvious to say we’ve tested multiple vehicles on the same track performing the same manoeuvres at 18C, 19C, 20C etc. But we’d never before been able to leverage that. The way AI makes predictions by calibrating historical data is very logical.”
Track testing data optimisation through AI
Just about every automotive OEM is going through some form of digital transformation right now.
Whilst the goals differ slightly, increasing the proportion of development work that occurs digitally rather than physically testing is a recurring theme.
Maybe now is the time to run some tests and experiments to see how AI can impact the way you do track testing.